SDSS spectra ExamplesΒΆ
Figure 7.1
A sample of 15 galaxy spectra selected from the SDSS spectroscopic data set
(see Section 1.5.5). These spectra span a range of galaxy types, from
star-forming to passive galaxies. Each spectrum has been shifted to its rest
frame and covers the wavelength interval 3000-8000 Angstroms. The specific
fluxes, , on the ordinate axes have an arbitrary
scaling.
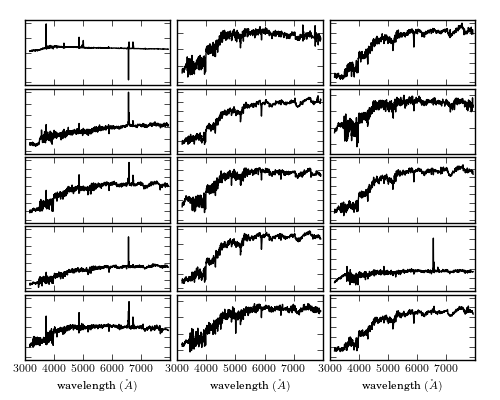
# Author: Jake VanderPlas
# License: BSD
# The figure produced by this code is published in the textbook
# "Statistics, Data Mining, and Machine Learning in Astronomy" (2013)
# For more information, see http://astroML.github.com
# To report a bug or issue, use the following forum:
# https://groups.google.com/forum/#!forum/astroml-general
import numpy as np
from matplotlib import pyplot as plt
from sklearn.decomposition import RandomizedPCA
from astroML.datasets import sdss_corrected_spectra
#----------------------------------------------------------------------
# This function adjusts matplotlib settings for a uniform feel in the textbook.
# Note that with usetex=True, fonts are rendered with LaTeX. This may
# result in an error if LaTeX is not installed on your system. In that case,
# you can set usetex to False.
from astroML.plotting import setup_text_plots
setup_text_plots(fontsize=8, usetex=True)
#----------------------------------------------------------------------
# Use pre-computed PCA to reconstruct spectra
data = sdss_corrected_spectra.fetch_sdss_corrected_spectra()
spectra = sdss_corrected_spectra.reconstruct_spectra(data)
lam = sdss_corrected_spectra.compute_wavelengths(data)
#------------------------------------------------------------
# select random spectra
np.random.seed(5)
nrows = 5
ncols = 3
ind = np.random.randint(spectra.shape[0], size=nrows * ncols)
spec_sample = spectra[ind]
#----------------------------------------------------------------------
# Plot the results
fig = plt.figure(figsize=(5, 4))
fig.subplots_adjust(left=0.05, right=0.95, wspace=0.05,
bottom=0.1, top=0.95, hspace=0.05)
for i in range(ncols):
for j in range(nrows):
ax = fig.add_subplot(nrows, ncols, ncols * j + 1 + i)
ax.plot(lam, spec_sample[ncols * j + i], '-k', lw=1)
ax.yaxis.set_major_formatter(plt.NullFormatter())
ax.xaxis.set_major_locator(plt.MultipleLocator(1000))
if j < nrows - 1:
ax.xaxis.set_major_formatter(plt.NullFormatter())
else:
plt.xlabel(r'wavelength $(\AA)$')
ax.set_xlim(3000, 7999)
ylim = ax.get_ylim()
dy = 0.05 * (ylim[1] - ylim[0])
ax.set_ylim(ylim[0] - dy, ylim[1] + dy)
plt.show()